一些簡單的凸分析及其应用
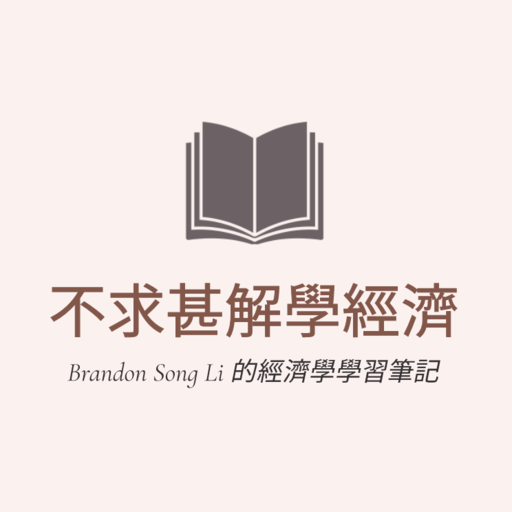
一些簡單的凸分析及其应用
时隔两年多,恢复更新了。这是一些簡單的凸分析的续篇。
目前只是草稿,会有很多错误。
Don’t follow my arguments for now, it’s just a draft of a draft.
在这篇中,我们要得到高微(一)中消费者理论和厂商理论的一些性质,比如关于支出函数、成本函数和利润函数。具体地说,我们的目标是得到 conjugate duality theorem 和 support function theorem。我们将先从 indicator function 开始,然后是 conjugate,随后引入 support function,作为 concave/convex indicator function 的 conjugate,之后是 Young-Fenchel conjugate theorem 和 Legendra transformation。
The reason for taking these notes is that we neither need to nor should we memorize the properties of the expenditure, profit, and cost functions by rote, since they are unified as direct applications of the support function theorem: they’ve just been attached names under scienarios with different economic interpretations. (Although for indirect utility function, things are tricky)
一些簡單的凸分析及其应用
The support function theorem
Let be a convex set in and let be the concave indicator function of , i.e.
if then ; if then (for a convex indicator function, then if then , but always for a convex set, and there is no such a notion as “concave set”)
Because the punishment is to an infinite extent, it should satisfy the constraint.
And let be the associated support function, i.e. .
which is, for a given ,
choose such that as small as possible. since we know that whenever , then , so is positive infinity, while for then , so to get the infimum, we must choose from , in this case, the infimum of is equivalent to the infimum of . We can interprate it this way: we are minimizing a linear function (e.g. choosing quantities vector, facing price vector, with a constraint that we must choose from the convex set , such as the upper contour set of a quasi-concave utility function; or the upper contour set of the production function, although when we interprate it as a cost function the here is actually the and should not be confused with the price of production)
and let be the set of solutions to (if any. interpretation: the cost minimizing factor demand with the requirement of producing at least as a level of producing some , or the expenditure minimizing good demand with the requirement of generating at least as a level of some utility level ). Then
- (1) the support function is concave in
(interpretation: the expenditure function, i.e. already substituting into the optimal solution; the cost function, also already substituting the optimal solution. So it is the value function)
(i.e. increasing price will increase cost/expenditure, but in a slower and slower speed),
upper semicontinuous, and homogeneous of degree one.
f is continuous on the interior of its domain, and weakly increasing (or some people say non-decreasing) in (increasing factor price will never reduce cost; increasing goods price will never reduce expenditure)
-
(2) if is closed and convex, then the conjugate of the conjugate gives us back to the concave indicator function, which is . Therefore, and . i.e. We can recover the upper coutour set from our support function (expenditure function, cost function,…).
-
(3) if is convex, the minimizer satisfies
-
(4) if for , then .
-
(5) is homogeneous of degree zero. (the factor demand, the Hicksian demand)
-
(6) if is convex and is second order continuously differentiable, then is differentiable and the matrix is symmetric and negative semi-definite. Moreover,
The Conjugate Duality Theorem
suppose is proper, concave and upper semi-continuous. Then the following are equivalent:
-
(1) minimizes
-
(2)
-
(5)
-
(6) minimizes
Attaching names
The properties of consumer expenditure function
-
(i) [support function theorem (1)] the support function is concave in , which is is concave in .
-
(ii) [support function theorem (1)] the support function is homogenous of degree one in , which is is homogeneous of degree one in .
-
(iii) [support function theorem (1)] the support function is continuous on the interior of its domain, so we have to tackle with the interior domian thing so that the support function is not only upper semi-continuous, and to interprate continuous in and .
-
(iv) [support function theorem (1)] the support function is weakly increasing (or some people say non-decreasing) in which is is nondecreasing in for any k. So we have to interprate to make it strictly increasing in as well.
-
Shepard’s lemma: [support function theorem (3)] if is convex, the minimizer satisfies
-
[support function theorem (4)] law of demand for Hicksian demand
-
[support function theorem (5)] demand is homogenous of degree zero.
-
[support function theorem (6)] the Slutsky Matrix
The properties of the cost fuction
- (i) [support function theorem (1)] the support function is concave in , which is is concave in .
Homogenous of degree one in p.
- (ii) [support function theorem (1)] the support function is homogenous of degree one in , which is is homogenenous of degree one in .
strictly increasing in u and nondecreasing in for any k.
-
(iii)
-
(iv)
-
Shepard’s lemma: [support function theorem (3)] if is convex, the minimizer satisfies
-
[support function theorem (4)] law of factor demand
The properties of the profit fuction
with additional reformulation, from to
-
(i) homogeneous of degree one in p and w.
-
(ii) in p and in w.
-
(iii) convex in p and w.
- (iv) is convex in p, and .
recoverability
support function theorem (2)
references
- KC Border’s Notes. (Of course, it’s always KC Border)
- Nam Nguyen’s course
- 標題: 一些簡單的凸分析及其应用
- 作者: (Brandon) Song Li
- 撰寫于 : 2024-11-08 00:06:33
- 更新于 : 2025-01-25 05:24:57
- 連結: https://brandonsli.com/p/9b881b90.html
- 版權宣告: 保留所有權利 © (Brandon) Song Li